An article by Dr. Dennis Proppe, Co-Founder & CAIO CrossEngage
“Silver Bullet AI”
Customer-centricity is the current mantra of existing customer marketing. Achieving this goal requires massive efforts for companies, especially in the B2C sector and for companies with large customer bases. In these areas, there are usually hundreds of thousands to many millions of customers with whom individual communication is to be optimized in terms of revenue. This cannot be done with manual processes. Most companies therefore rely on marketing automation tools, which are then controlled with a very, very large number of complex rules. These rules are usually set up by hand, often as a consequence of manual BI analyses.
In the long run, this only works to a limited extent: A normal retail company has hundreds of touchpoints with its customers, on many channels in the most diverse situations. In addition, there is very strong competition from platforms such as Amazon, Zalando or Otto, which own massive amounts of data and can therefore respond very individually to customers in their specific situations. In such an environment, once created, rigid rules such as “If the customer does not complete his shopping cart, wait twelve hours and then send a retargeting with a ten-Euro-voucher” are no longer sufficient to survive in competition.
At this moment, the “silver bullet AI” often appears on the scene. Driven by providers, but also by their own “willing to believe”, the machine learning techniques underlying AI are then often seen as the salvation, guaranteeing a fast and successful transformation to fully automated customer-centricity.
In the reality experienced by the author for over ten years, this moment actually tends to trigger a long and complex transformation process that carries a high risk of failure, but can also have an absolute positively disruptive impact on the company. In the following, we describe the typical six phases of such a process and their core characteristics. The author has accompanied about 100 such transformations at CrossEngage (former Gpredictive) and therefore draws on rich practical (and sometimes painful) experience.
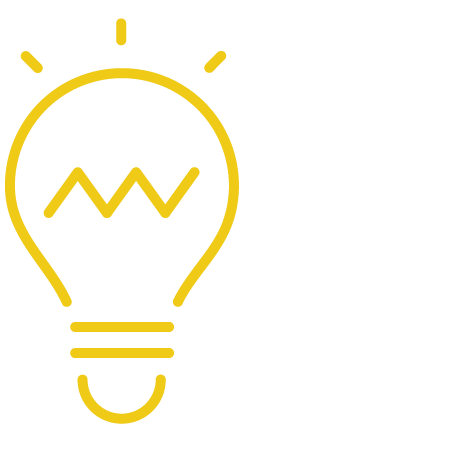
Phase 1: Inception of An Idea
This phase marks the first exposure to artificial intelligence and machine learning methods in the field of existing customer marketing. Companies in this phase are often overwhelmed by the possibilities and efforts of modern existing customer marketing. At this stage, campaigns are often put together from scratch and a lot of manual analysis is done. There is a prevailing feeling of not having everything under control and being very far from a coherent, customized marketing program. In this situation, the emergence of potential solutions that independently create custom decision rules from automated analytics is often the “aha” moment making business leaders recognize AI as a potential solution to the complexity problem.
Phase 2: Fear of missing out
This phase is often driven by a perceived external pressure from market companions, solution providers, media and advisors. Very often, artificial intelligence is touted as the solution to many problems in operational marketing facing existing customers. Likewise, adoption by other companies is perceived to be very advanced here. The bottom line is that there is a strong sense of time pressure at the executive level, plus the impression of artificial intelligence as a silver bullet. Accordingly, there is a big pull to adopt AI.
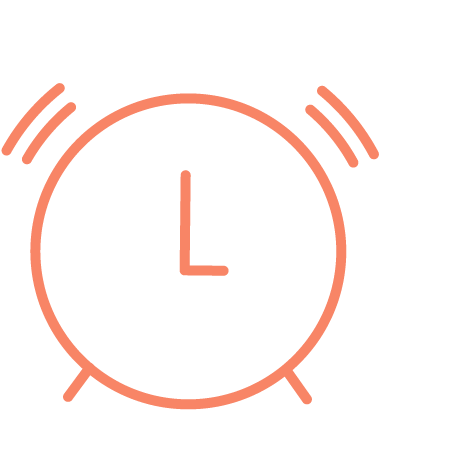
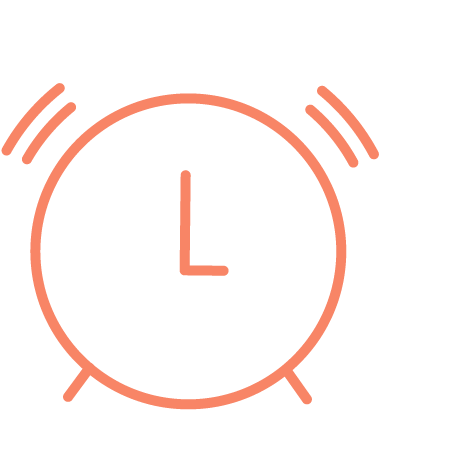
Phase 2: Fear of missing out
This phase is often driven by a perceived external pressure from market companions, solution providers, media and advisors. Very often, artificial intelligence is touted as the solution to many problems in operational marketing facing existing customers. Likewise, adoption by other companies is perceived to be very advanced here. The bottom line is that there is a strong sense of time pressure at the executive level, plus the impression of artificial intelligence as a silver bullet. Accordingly, there is a big pull to adopt AI.

Phase 3: Touching Ground
As a result, initial efforts are often made without a clear strategy. This is the great hour of proof-of-concepts. These realize typical, generic machine learning use cases where implementation is easy and fast. Unfortunately, at this stage, there is often no actual alignment with the real business goals and the impact of the methods deployed. This leads to highly inflated expectations that are then disappointed after the initial project phase. Hitting on the ground of reality then results in a company-internal AI winter and many companies drop out at this stage, annoyed and disillusioned.
Phase 4: Light at The End of The Tunnel
Companies that continue despite initial negative experiences eventually reach the point where they can see how truly customer-centric marketing can deliver massive ROI for the first time. Often, the initial feedback from smaller test campaigns leads to this. This feeling often appears nine to twelve months after the start of the project, when the roughest data issues have been solved and a real strategy has emerged through a lot of internal communication and engagement. For the first time, there is a framework against which success can be measured in a resilient way. At this stage, there are not yet any reliable ROI results, but companies that get this far usually do not give up.
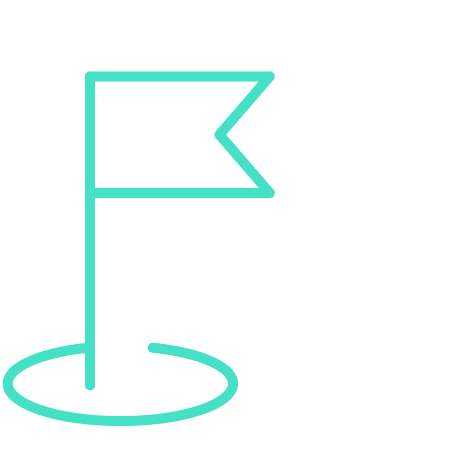
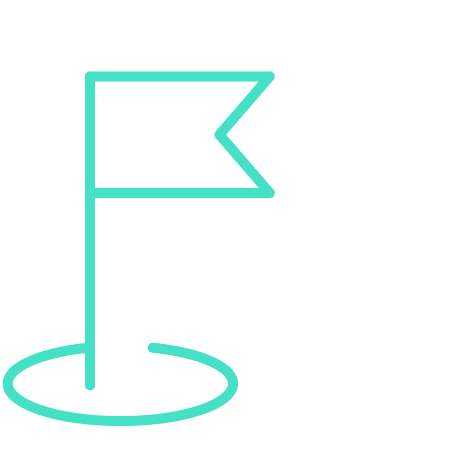
Phase 4: Light at The End of The Tunnel
Companies that continue despite initial negative experiences eventually reach the point where they can see how truly customer-centric marketing can deliver massive ROI for the first time. Often, the initial feedback from smaller test campaigns leads to this. This feeling often appears nine to twelve months after the start of the project, when the roughest data issues have been solved and a real strategy has emerged through a lot of internal communication and engagement. For the first time, there is a framework against which success can be measured in a resilient way. At this stage, there are not yet any reliable ROI results, but companies that get this far usually do not give up.
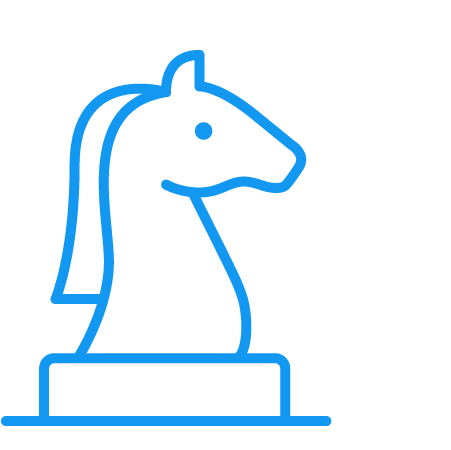
Phase 5: Getting The First Really Good Results
At some point it happens: After a few test campaigns with a wide variety of use cases, there is one action, one machine learning model that stands out. This can be the more efficient handling of customers who are at risk of becoming inactive, or the accurate tracking of new buyers who have a high future potential. The results are astounding, at times almost unbelievably good. At that moment, the whole organization realizes the potential of the whole initiative and a very, very strong pull from different sides starts. Now everyone wants to benefit from this new opportunity. In the worst case, marketers then try to satisfy all requests right away. This results in mistakes, which in turn can lead to poor results. So this phase once again involves the risk of a setback.
Phase 6: Automate The Process to Ensure Steady & Great Results
In the best case, the company is now moving forward again in a planned, patient manner and with a clear strategy. The faced use cases are gradually rolled out, and experiments are separated from ongoing optimizations. With a clear focus on automated processes, single parts of the customer lifecycle are optimized automatically. In its final stage of expansion, such a system can make many small day-to-day decisions for customer communications and incentive management per day, generating incremental ROI with each decision. Due to the sheer mass of decisions made possible by automation, these optimizations add up massively.
This process often results in further restructuring processes in customer relationship management tools. The automated exchange of data and forecasts is recognized as a decisive success factor. Seamless exchange with a customer data platform (CDP) is recommended in order to provide bundled data for forecasts and to be able to automatically send out CRM measures across all channels. Tools of this type bundle all data sources in comprehensive customer profiles and enable the customer-specific delivery of campaigns across different channels.


Phase 6: Automate The Process to Ensure Steady & Great Results
In the best case, the company is now moving forward again in a planned, patient manner and with a clear strategy. The faced use cases are gradually rolled out, and experiments are separated from ongoing optimizations. With a clear focus on automated processes, single parts of the customer lifecycle are optimized automatically. In its final stage of expansion, such a system can make many small day-to-day decisions for customer communications and incentive management per day, generating incremental ROI with each decision. Due to the sheer mass of decisions made possible by automation, these optimizations add up massively.
This process often results in further restructuring processes in customer relationship management tools. The automated exchange of data and forecasts is recognized as a decisive success factor. Seamless exchange with a customer data platform (CDP) is recommended in order to provide bundled data for forecasts and to be able to automatically send out CRM measures across all channels. Tools of this type bundle all data sources in comprehensive customer profiles and enable the customer-specific delivery of campaigns across different channels.
Now Readers May Think, “Good to Know, but What Can I Learn from It?”
In general, as a company, you have the chance to anticipate things by recognizing positive and negative patterns in processes and thus proactively defuse them. All in all, on the way to customer-centricity, you should above all try to reduce the size of swings. Instead of always swaying between being sky-high and saddened to death in the project team, rigorous expectation management is crucial from phase 1 onward. All parties involved must be aware that there will be positive and negative phases. It should also be clear that such a process will take a lot of time, money, and internal resources. The transformation to a truly customer-driven company is not a spontaneous revolution, but an evolution planned for the long term and carried out with a cool head.
In order to be able to develop this staying power, it is very important to measure all effects cleanly from the very beginning and to repeatedly test the status quo against the new ideas and processes. This is the only way to automate decisions step by step along the way. The best successes are achieved by organizations that constantly test and consistently use control groups and question the status quo.
The sole driver of the agenda should be the company itself: Especially in the second phase, marketers must not be guided by sellers or herd drive. The transformation to customer-centricity is not a sprint, but a long-distance run, so the winner is not always the one who starts running the fastest at the beginning, but the one who actually gets there in the end.
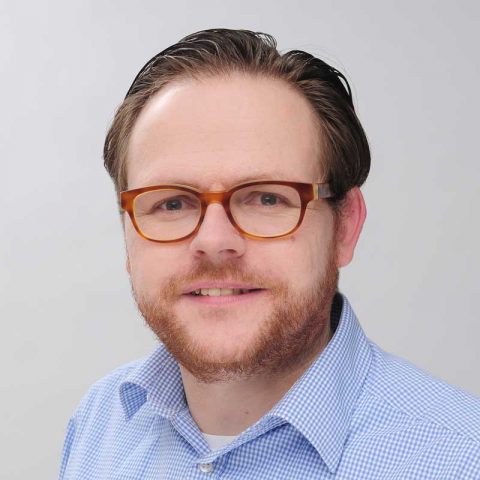
About the author: Dr. Dennis Proppe is Chief AI Officer at CrossEngage and responsible for product development and vision execution. He has 15 years of experience in machine learning and has been building AI and engineering teams for ten years. Dennis holds a PhD in Marketing and Statistics from Kiel University.